How are advancements in machine learning algorithms enhancing predictive analytics in sectors like finance and healthcare?
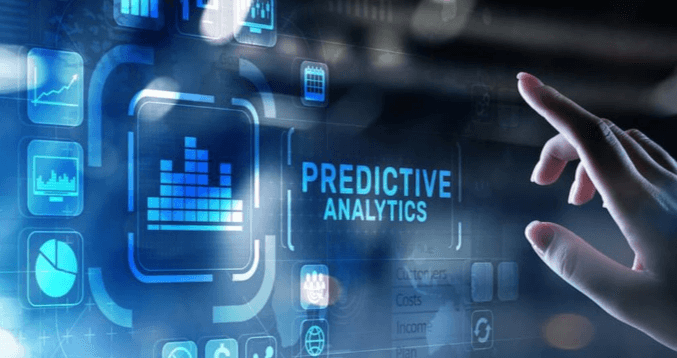
Predictive analytics is at the forefront of technological advancements, particularly in sectors like finance and healthcare. With the rapid evolution of machine learning algorithms, these industries are witnessing unprecedented improvements in their ability to forecast outcomes, manage risks, and optimize operations. This article delves into the transformative impact of machine learning on predictive analytics, highlighting key developments and their implications.
Introduction
Predictive analytics has become an indispensable tool for various industries, enabling them to anticipate trends, make informed decisions, and gain a competitive edge. At the heart of this capability lies machine learning—a subset of artificial intelligence that focuses on building systems that learn from data and improve their performance over time. This technology has revolutionized the way organizations approach predictive analytics, especially in critical sectors like finance and healthcare.
The Role of Machine Learning in Predictive Analytics
Machine learning algorithms have significantly enhanced the precision and reliability of predictive models. These algorithms can analyze vast amounts of data, identify complex patterns, and make accurate predictions with minimal human intervention. In finance, this translates to better risk management and investment strategies, while in healthcare, it leads to improved patient outcomes and operational efficiency.
Machine Learning Algorithms Driving Predictive Analytics
Several advanced machine learning algorithms are driving the success of predictive analytics:
- Regression Algorithms: These are used to predict a continuous outcome based on input variables. Linear regression, polynomial regression, and support vector regression are commonly used in finance for predicting stock prices and in healthcare for forecasting disease progression.
- Classification Algorithms: These algorithms classify data into predefined categories. Logistic regression, decision trees, random forests, and support vector machines are pivotal in both sectors for fraud detection in finance and diagnosis in healthcare.
- Clustering Algorithms: Used to group similar data points, clustering algorithms like k-means and hierarchical clustering help in segmenting customers in finance and identifying patient subgroups in healthcare.
- Neural Networks: Deep learning models, particularly neural networks, have shown exceptional performance in predictive analytics. Convolutional neural networks (CNNs) and recurrent neural networks (RNNs) are extensively used for image and sequence data, respectively.
- Ensemble Methods: Techniques like boosting, bagging, and stacking combine multiple models to improve prediction accuracy. These methods are highly effective in both finance and healthcare for aggregating diverse data sources and models.
Risk Management and Fraud Detection
Machine learning algorithms have drastically improved risk management in finance. Predictive models can now assess the likelihood of loan defaults, enabling banks to make informed lending decisions. Additionally, fraud detection systems leverage machine learning to analyze transaction patterns and identify anomalies in real-time, reducing financial losses and enhancing security.
Algorithmic Trading
In the realm of trading, machine learning models analyze historical data to identify profitable trading opportunities. These algorithms can execute trades at optimal times, maximizing returns and minimizing risks. High-frequency trading firms rely heavily on these models to stay ahead in the competitive market.
Customer Insights and Personalization
Financial institutions use predictive analytics to gain deep insights into customer behavior. By analyzing transaction data, machine learning models can predict customer needs and preferences, allowing for personalized product offerings and improved customer service.
Early Disease Detection and Diagnosis
Machine learning algorithms have revolutionized disease detection and diagnosis. Predictive models can analyze medical images, genetic data, and electronic health records to identify early signs of diseases such as cancer, diabetes, and heart conditions. This early detection is crucial for timely intervention and improved patient outcomes.
Personalized Treatment Plans
Predictive analytics in healthcare enables the development of personalized treatment plans. By analyzing patient data, machine learning models can predict how patients will respond to different treatments, allowing healthcare providers to tailor interventions to individual needs.
Operational Efficiency
Hospitals and clinics leverage predictive analytics to optimize their operations. Machine learning models can forecast patient admissions, manage staff schedules, and predict equipment maintenance needs, ensuring smooth and efficient healthcare delivery.
Challenges and Considerations
While the advancements in machine learning and predictive analytics are impressive, there are challenges to address. Data privacy and security are paramount, especially in healthcare where patient information is sensitive. Ensuring the ethical use of machine learning models and mitigating biases in data are also critical considerations.
Future Prospects
The future of predictive analytics, powered by machine learning, is promising. In finance, we can expect more sophisticated models that offer even greater accuracy and insights. In healthcare, advancements in genomics and wearable technology will provide more data for predictive models, leading to even more personalized and effective treatments.
FAQs
How is machine learning used in predictive analytics?
Machine learning is used in predictive analytics to analyze large datasets, identify patterns, and make accurate predictions with minimal human intervention. It enhances the precision and reliability of predictive models across various industries.
What are some common machine learning algorithms used in predictive analytics?
Common machine learning algorithms used in predictive analytics include regression algorithms, classification algorithms, clustering algorithms, neural networks, and ensemble methods.
How has machine learning improved risk management in finance?
Machine learning has improved risk management in finance by enabling more accurate predictions of loan defaults and enhancing fraud detection through the analysis of transaction patterns and identification of anomalies.
How is predictive analytics used in healthcare for early disease detection?
Predictive analytics in healthcare uses machine learning models to analyze medical images, genetic data, and electronic health records to identify early signs of diseases, allowing for timely intervention and improved patient outcomes.
What are the ethical considerations in using machine learning for predictive analytics?
Ethical considerations include ensuring data privacy and security, mitigating biases in data, and ensuring the ethical use of machine learning models to avoid unintended consequences.
What is the future of predictive analytics in finance and healthcare?
The future of predictive analytics in finance and healthcare is promising, with expectations of more sophisticated models offering greater accuracy and insights, and advancements in genomics and wearable technology providing more data for personalized treatments.
Conclusion
Advancements in machine learning algorithms are significantly enhancing predictive analytics in finance and healthcare. These technologies enable more accurate predictions, better risk management, and improved patient outcomes. As machine learning continues to evolve, its impact on predictive analytics will only grow, offering even more transformative benefits across various sectors.